We use the solution for purchase accounting, where we need a lot of invoices from various vendors.
CEO at a outsourcing company with 1,001-5,000 employees
Used for purchase accounting and to receive a lot of invoices from various vendors
Pros and Cons
- "The solution allows us to continue with vendors whose information comes in correctly and to stop the automation for vendors with many items that are not clearly defined."
- "Sometimes, when the number of items is very large, the solution doesn't properly identify them."
What is our primary use case?
What is most valuable?
The solution allows us to continue with vendors whose information comes in correctly and to stop the automation for vendors with many items that are not clearly defined. The precision is not very high when there is a lot of text in the item table.
What needs improvement?
Sometimes, when the number of items is very large, the solution doesn't properly identify them. It's not 100% accurate, but it gives an 85% to 90% output. The solution doesn't work properly when too much data is on the table. Using 10 pages of data only for tables makes it difficult to collate the data.
We would like to have a better conversion of data, which is in tables and available on multiple pages. The same table is repeated with different items across multiple pages, which is challenging. We have to give similar keywords when indicating the second or third page. We should be able to use the same keyword for one document to identify the first page. From the second page onwards, the keyword should be done away with.
The repeated keywords on the second and third pages create problems because not many keywords are available on the second and third pages. It is a simple table-to-table repetition. The keywords, including the vendor's registration number, address, phone number, or email, are unavailable on the table pages.
Pages 2 to 10 are all just simple tables. The keywords are not available on those pages, and that's where the system gets more complicated.
For how long have I used the solution?
I have been using UiPath Document Understanding for 3 years.
Buyer's Guide
UiPath Document Understanding
April 2024
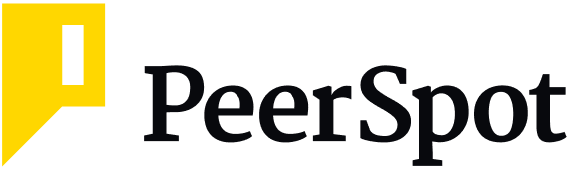
Learn what your peers think about UiPath Document Understanding. Get advice and tips from experienced pros sharing their opinions. Updated: April 2024.
771,170 professionals have used our research since 2012.
How was the initial setup?
A person who knows the system can deploy it very easily and quickly without any difficulty or complication.
What about the implementation team?
We take about 2 to 3 days to set up UiPath and install the UiPath Document Understanding programs we have developed. It takes time to create the vendor data and train the bot for each vendor. We spend about 40 minutes training the bot for each vendor.
What was our ROI?
The solution is a good investment. The investment of one year's license fees and development costs could be recovered in four to five months' time.
What's my experience with pricing, setup cost, and licensing?
The product is not very costly in itself. It is part of the normal license. The solution’s cost increases for machine learning or artificial intelligence because we have to go for UiPath Orchestrator. UiPath Orchestrator's cost is very high, around $10,000 per year.
What other advice do I have?
If somebody has a lot of vendors and about 100 invoices per day, we have to train the bot for various formats. We convert data from scanned PDF into a standard Excel table, including invoice numbers, purchasing order numbers, dates, and vendor names. The solution uses typed or printed data, not handwritten. It's difficult to capture handwritten data because different types of handwriting increase the errors in capturing the data.
About 80% of our organization’s documents are completely processed automatically. Sometimes, we don't have good data capture when the PDF is not of good quality. You need to have good-quality scans. Using the artificial intelligence or machine learning capabilities of UiPath Document Understanding is costly.
Integrating the solution with other ERP systems and applications is very easy. There are a lot of commands specifically for SAP. Initially, almost every document was verified by human beings. After gaining confidence and segregating the vendors who were giving out correct results, we stopped human intervention and started running on the bot completely.
When there were about 200 invoices per day, we employed about 5people to process the invoices manually in the ERP. When we introduced the UiPath Document Understanding bot, it could process faster and reduce the number of people required from 5 to 2. The solution helped reduce human errors and made processing in time possible, thereby avoiding delays.
Users should take the solution as an opportunity to reduce costs and not expect 100% results. Expecting 100% results would make it a failure from day 1 because it will not give 100% results. It will give you 80% accurate and 20% jumbled details because of the various complications arising from lengthy or unclear documents. If your expectation is reasonable, your success rate will be high.
Overall, I rate the solution an 8 out of 10.
Disclosure: My company has a business relationship with this vendor other than being a customer: Implementor
Last updated: Apr 24, 2024
Flag as inappropriateCEO and Founder at SyncIQ
Helps to reduce human error, and fully automate 95 percent of processes, but the price is high
Pros and Cons
- "The most valuable feature is key-value pair and table extraction."
- "The UiPath APIs lack reliable table parsing."
What is our primary use case?
Our primary clients are in the pharmaceutical and hospitality sectors. We recently developed a process using UiPath Document Understanding called 'Medicaid automation' to automatically download invoices and structured data from legacy systems. We then built an ETL pipeline to further process this information. Additionally, we have experience automating contract downloads and parsing data from contracts, even for structured data sources.
Automating processes using structured data is straightforward. However, in many cases, we need to involve human workers because data extraction is not very accurate. Therefore, we need a solution to integrate human input and structured data into the automation pipeline to minimize manual intervention. Additionally, when accuracy requirements are very high, we can also set up a user interface. Conversely, for less stringent accuracy requirements, we can create a fully automated pipeline. This is the core idea behind using UiPath Document Understanding. We aim to automate processes for functions like finance, resource management, and revenue management.
How has it helped my organization?
I work primarily in the pharmaceutical and hospitality industries. Within these industries, specific domains have different usage requirements. For example, in the pharmaceutical industry, I work with finance teams, and their focus on unstructured data includes tasks like invoice processing. Revenue management teams might leverage unstructured data for contract management, extracting key details for further use. Both finance and revenue management teams should consider how generative AI technology can streamline their workflows. In my experience, I've implemented an agent capable of extracting data from compliance documents and providing structured responses to users. Other use cases involved HR-related document queries and automated responses. Within the hospitality sector, I've worked on customer success and revenue management projects. On the customer success side, unstructured data related to loyalty programs could be analyzed for insights. We also explored automating email generation and streamlining tasks related to standard operating procedures. Revenue management in hospitality often involves contract automation. For a large hospitality company, I worked on a project to extract data from B2B contracts stored in Salesforce, pushing that information directly into their financial system. It's important to note that while I used unstructured documents as a foundation for these projects, not all of them specifically employed UiPath.
Using UiPath Document Understanding, we have successfully processed invoice documents and contracts. We are now expanding to handle various additional contract types based on specific use cases. This could involve rebate management, B2B interactions, or other scenarios. Additionally, we can handle other document types, such as per-case order documents and various SOP documents (compliance and operational). Finally, we have also explored applying Document Understanding to marketing materials related to sales rep automation, where product information can be leveraged to generate responses.
We use UiPath Document Understanding for many formats. The format of documents depends on their type. Invoices and purchase orders, for example, are considered semi-structured. This means they contain a combination of elements, such as tables, key-value pairs, and line items, but these elements can exist in different templates and with some variation between vendors. Contracts, on the other hand, are largely unstructured. While they may contain structured elements like tables, they also often include running text and information that is difficult to categorize in a predefined format.
We can fully automate the process for 95 percent of the documents. The more high-risk financial documents may need human intervention.
AI capabilities significantly reduce development effort for handling encrypted data while simultaneously increasing its overall scope. This allows me to achieve what was previously impossible with conventional APIs, even in advanced tools like UiPath. While UiPath also utilizes a broad model for data extraction, they are now expanding towards generative AI. Consequently, we benefit from improved extraction quality and the ability to extract data in the desired structure, all with minimal development effort thanks to AI.
When human validation is required, it takes one to two minutes for a five-page document.
Previously, reviewing a difficult document like a contract could take around 30 minutes, while an easier document like an invoice took 10-15 minutes. After automation, processing invoices got significantly faster, taking less than half a minute. This is because the complexity of invoices is generally lower compared to contracts. For contracts, automation was reduced to around three minutes. In simpler cases, the processing time could even be reduced to as low as one to 15 seconds.
The significant reduction in processing time leads to a notable decrease in human errors.
Our clients can see the time to value within the first three months.
What is most valuable?
The most valuable feature is key-value pair and table extraction. While we previously relied on UiPath and Amazon APIs, we've transitioned to generative AI for its superior performance on unstructured data. However, this shift presents a challenge: while UiPath and Amazon provided consistent output and value, generative AI outputs can vary significantly across different documents. This means we still need logic-based parsing for tables, even though they often share similar formats.
What needs improvement?
The UiPath APIs lack reliable table parsing.
The accuracy of document extraction depends on the document's original format. For rich text documents, the accuracy is generally good. However, scanned documents like PDFs or images present a challenge and often yield lower accuracy. Another challenge arises when dealing with multiple documents in a single image. This scenario is common in invoice automation, where a single image might contain several invoices. Furthermore, processing files containing multiple document types, such as multiple invoices in one file, can be problematic. Currently, the system assumes each uploaded file represents a single document or invoice, which is not always the case. To address these challenges, I propose enhancing UiPath Document Understanding to analyze the entire document, not just individual pages. This would allow the system to identify individual invoices within a multi-page document and assign extracted data to the corresponding invoice.
I would like custom key value integration instead of generic key values for extraction.
The cost of UiPath Document Understanding has room for improvement.
For how long have I used the solution?
I have been using UiPath Document Understanding and other IDP products/APIs for four years.
What do I think about the stability of the solution?
UiPath Document Understanding is generally considered a stable product. If we encounter issues when using it in the context of a complex backend process, the problem is likely not with UiPath itself but rather with the specific process design and the components involved in its development.
What do I think about the scalability of the solution?
The high cost of adding bots hinders our ability to scale UiPath Document Understanding.
How was the initial setup?
The deployment takes around five days for my team to complete.
What's my experience with pricing, setup cost, and licensing?
UiPath Document Understanding carries a premium price tag, but its current technological capabilities may not yet fully justify the cost.
What other advice do I have?
I would rate UiPath Document Understanding five out of ten.
UiPath Document Understanding requires significant ongoing maintenance, especially when it integrates with screens or utilizes user interface automation. This is because changes to the website structure are highly likely to cause these integrations to break. Backend automation, on the other hand, typically requires less ongoing maintenance. However, it is still recommended to dedicate resources to monitor the solution approximately 50 percent of the time. This proactive approach helps ensure uninterrupted business processes even after a proper initial development phase.
For automating cloud-native platforms, scripting often proves to be a more suitable approach compared to tools like UiPath. However, when dealing with legacy systems, UiPath might offer a more effective solution.
Which deployment model are you using for this solution?
Private Cloud
Disclosure: My company has a business relationship with this vendor other than being a customer: Consultant
Last updated: Mar 4, 2024
Flag as inappropriateBuyer's Guide
UiPath Document Understanding
April 2024
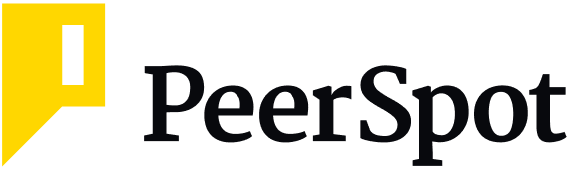
Learn what your peers think about UiPath Document Understanding. Get advice and tips from experienced pros sharing their opinions. Updated: April 2024.
771,170 professionals have used our research since 2012.
Owner at Orange Horse
Can understand varying document formats, provides efficient integration, and saves manual effort
Pros and Cons
- "The quality of the input documents is crucial because sometimes healthcare providers prefer automated processing rather than human review."
- "The results of classifying patient documents within UiPath Document Understanding need to be more accurate."
What is our primary use case?
In a medical healthcare department, when we need to retrieve digital documents, we need to classify them. The first step is to use AI to understand what type of documents we're dealing with. Once we've identified the template, we can extract information using specific OCR tools. Depending on the confidence of the extracted results, we may need to apply additional OCR, use a more active tool, or pass the document to an agent for review if the AI doesn't recognize a specific element like the "person page of the commission." Finally, the extracted fields are classified within the system and organized into different folders. This is the process I'm using with UiPath Document Understanding.
How has it helped my organization?
Document Understanding can complete each document within one second.
It can be applied to the healthcare industry to streamline the processing of medical documents. This includes scanning and applying OCR to convert physical documents into digital formats.
We can tune the AI component to improve the quality and accuracy of the documents being processed.
Typically, the AI process involves several steps. Firstly, it recognizes the template, which essentially identifies the input format being used. Secondly, it applies rules configured in a JSON file. This file specifies details like the expected fields for the recognized template, such as name, age, date of birth, and security address. The AI then reads and analyzes data from the specified location based on the recognized template. It applies the predefined rules to extract relevant information and search for the required fields. If the input doesn't match any known template, it employs more general search methods to locate the desired information. This is the core functionality of the internal AI component.
Of the 1,000 documents we process, 90 percent are completely automated.
My three OCR tools each incorporate three AI components. These components work in tandem, with the activity determining which AI component takes the lead. For example, if the first AI requires a minimum accuracy of 86 percent and encounters text with 85 percent accuracy, it passes the task to the next AI component. This next component employs a different OCR tool in an attempt to achieve the required accuracy. If it still falls short, the task is then routed to a human agent.
Our integrations leverage robust API connection services. A single, secure authentication method protects access to JSON files. Requests are sent and product responses are seamlessly handled. This API-based approach provides faster and more efficient integration compared to manual interface interactions.
UiPath now includes a document understanding AI components, eliminating the need for third-party solutions like ABBYY. This allows for quick and automated extraction, analysis, and template recognition of information from various documents. By training the system with diverse examples, the AI component can become highly efficient, similar to ABBYY's global OCR capabilities. This is a significant improvement, as it eliminates the need for additional integrations like ABBYY within UiPath projects.
I found UiPath Document Understandings' ability to understand varying document formats to be good. I had no issues with the templates I was using.
Using AI and machine learning can significantly speed up the recognition of new formats, templates, customers, or entities introduced into our process. It is particularly beneficial when dealing with low-quality documents, which often require manual intervention. By implementing a machine learning model at the beginning of the process, the system can learn from successful agent solutions and incorporate them into future scenarios. Clear feedback, including agent ID and task details, further enhances this learning process. As a result, machine learning can help save time, reduce costs, and improve overall process accuracy. This makes it a valuable tool within UiPath.
Less than ten percent of processed documents require human validation. However, when customers provide input that falls outside pre-defined templates the usual 90 percent of cases, the system cannot recognize it and fails to notify agents. This means a new template will be implemented to include human-agent collaboration when training AI models.
The validation process depends on the specific template and the data being acquired. If all data is extracted from the entire template, the validation process can take less than one minute.
The manual document process took us around ten minutes and now with UiPath Document Understanding, the process is within seconds.
Since implementation, human error has been reduced by 30%.
UiPath Document Understanding has helped save 50% of our time in instances when no human validation is required.
What is most valuable?
The quality of the input documents is crucial because sometimes healthcare providers prefer automated processing rather than human review. However, this preference depends on the complexity of the resolution required and the document type e.g., JPEG, TIFF. I find the quality of the input documents as the most valuable part of the automation.
What needs improvement?
At the end of the process, we classify documents in our external application, similar to a CRM system. This classification is based on the documents stored in the new system. The results of classifying patient documents within UiPath Document Understanding need to be more accurate.
For how long have I used the solution?
I have been using UiPath Document Understanding for three years.
How are customer service and support?
UiPath offers excellent technical support due to its high-tech nature and the complex needs of its customers. This support is crucial for several reasons. One such reason is the customer success plan, which provides dedicated API support and a specialist focused on existing customers. This fosters close communication between the customer and UiPath, facilitated by two individuals who actively monitor and manage the customer's needs every week.
How would you rate customer service and support?
Positive
Which solution did I use previously and why did I switch?
Previously, we used manual processes for all our tasks. We transitioned to UiPath Document Understanding due to its integration of AI components. It is more flexible to our needs.
What was our ROI?
We saw a return on investment within three months of deploying UiPath Document Understanding.
What's my experience with pricing, setup cost, and licensing?
The pricing structure is based on the number of robots installed. While a single robot may suffice for some customers, others may require more depending on their processing capacity needs and desired turnaround times.
The cost per license is significant, approaching ten thousand dollars. While not inexpensive, for high transaction volumes, the potential savings can be substantial.
What other advice do I have?
I rate UiPath Document Understanding an eight out of ten.
Disclosure: I am a real user, and this review is based on my own experience and opinions.
Last updated: Feb 22, 2024
Flag as inappropriateRPA Developer at Arkon Group LLC
Reduces human validation, offers good machine learning and has excellent document understanding
Pros and Cons
- "It's great for document understanding for invoices and installments."
- "It would be ideal if they could include more packages for more use cases."
What is our primary use case?
I've done multiple projects. A couple of them included invoice processing. It has a machine learning package that works out of the box. For invoices. I use that. It does a very good job.
I also use document understanding, which doesn't have any training. I trained it for the extraction of data for some forms like car loan installments. It did a pretty good job.
In addition, I used it for a medical department. I use document understanding.
How has it helped my organization?
We wanted to have a way to do data extraction from PDF documents. It helped us automate the process. For example, if you purchase a car, the loan installment paper includes items like the vehicle number, purchase information, buyer and seller information, et cetera. It can pull that out. We can also use it similarly in the healthcare industry, to get client details.
What is most valuable?
It's great for document understanding for invoices and installments.
When it comes to document understanding for handwriting, it does a decent job sometimes with handwriting, however, some people have weird handwriting and the OCR can struggle to pick up the information. In those cases, you have to read it yourself. However, overall, it does a decent job. I haven't used it to read checkboxes or bar codes. It works well with tables, however.
There are thousands of documents that are completely, automatically processed. It can process close to a few thousand invoices per day.
I also integrated it with the Action Center for some projects; It's pretty neat.
I like the machine learning skills and the fact that they come out of the box. They are packages that you can just deploy. The training of the ML is great; there is this tool that comes with it called Data Manager. That's very handy when you are labeling data and then using it.
The AI center is excellent. AI does a pretty good job covering all the needs that are needed for automating the process for semi-structured documents. The structured documents with the form extracted, overall, are pretty good. It's doing a very impressive job. I was surprised the first time I was exposed to it. Now, I actually enjoyed doing it. It allows me to automate items that are mundane. For example, if an employee is given a task to scrape data from invoices, which are PDFs, they can get the robot to do it. Due to the fact that the documents most of the time are semi-structured, machine learning can handle the task, and machine learning is doing a pretty good job of handling that instead of the employee.
I've used Forms AI. So far, my experience has been pretty good. That said, it only works for structured documents.
In terms of the documented understanding of integrating with other systems or applications, everything is good. You can integrate it with the action center, and it does a very good job. Everything is handy and easy to use. Integration overall is good.
Human validation is not always required for the outputs. It depends on the document. For invoices, you might need human validation 5% to 10% of the time. If it processes ten documents, I would expect one document at least to need human intervention. If you are building some custom ML skills for some documents, if the document itself is scanned well and positioned well, it does a pretty good job of extracting the needed fields. If it's slightly less quality then the robot will struggle with both the OCR or extracting and digitizing data. Overall, we might need 10% to 20% human validation. The validation process itself now takes about a minute with the help of automation. It's reduced everything by a minute or two to up to five or six minutes.
Document understanding has helped us to reduce human error by at least half.
What needs improvement?
The only problem that I can see with integration is some of the features cannot be used inside the loop. At least that was the case before. I don't know if they fixed it or not. You can't put some of the activities that are de-related inside the loop. It's going to throw an error if you do.
It would be ideal if they could include more packages for more use cases.
For how long have I used the solution?
I've used the solution for about a year.
How are customer service and support?
I've contacted technical support and they have been helpful.
How would you rate customer service and support?
Positive
What other advice do I have?
I'm a customer and end user. I work as a developer.
I'd rate the solution nine out of ten overall.
Disclosure: PeerSpot contacted the reviewer to collect the review and to validate authenticity. The reviewer was referred by the vendor, but the review is not subject to editing or approval by the vendor.
Last updated: Oct 15, 2023
Flag as inappropriateRPA Developer at Anza Business Services LLP
As we process more data, the solution adapts using machine learning to classify information more accurately
Pros and Cons
- "The validation process is easy. The Validation Station shows you the extracted data on one side and the document on the other, so you can easily scroll down and check if the data is accurate. You just need to click a checkbox. If you don't think it is fine, you have the option to add an exception. Based on that exception, you can create multiple conditions for how to address the same issue if it happens again."
- "I would like to see more integration of artificial intelligence. That's being implemented, but it would be a massive improvement to the solution's document processing. If UiPath achieves intelligent document processing, it will be far better than anything on the market. There are currently some limitations with the fields that could be addressed using a GPT engine. With an integrated AI model, you wouldn't need to create your taxonomy. You would only need to provide some prompts, such as "What is the property name?" It will store that as a variable."
What is our primary use case?
UiPath can handle normal, structured documents like forms and editable PDFs, but the data cannot be extracted from some unstructured documents with normal instructions. Non-standard documents are the most challenging thing for us. For example, let's say you have a hard copy of a receipt you get from a store, and you want to keep a record of it. You need to extract specific types of data and store it in Excel. Document Understanding can deal with these documents. You can configure it to scan the receipt and identify the data we're interested in.
We can provide a set of optimizations, classifications, and preconfigurations before we process the document. We created a taxonomy that we've predefined that these kinds of documents can conform to our security purposes. Using the taxonomy, Document Understanding can first classify the type of document, the arguments or variables we want to use, and the data we need to extract or store. Document Understanding can scan a written document and identify if a signature is present.
We keep a person in the loop in between because we can't 100 percent rely on the extraction. Document Understanding uses OCR which sometimes struggles with handwritten material. For example, it might mistake a six for a five. There must be a human in the loop to ensure quality. The device will send it to the validation station on your mobile phone. The bot will learn from the choices you make, and it will be more accurate the next time.
How has it helped my organization?
Document Understanding helps us to reduce human error. It can reduce the time staff spends on some tasks, but the amount of time saved depends on a few factors. We still need to validate the data because before proceeding, we sometimes collect and share sensitive data for our clients. We need a validation step in between to check before we send any data.
What is most valuable?
One benefit of Document Understanding is machine learning. As we process more data, we train Document Understanding to classify information more accurately. Document Understanding can extract and interpret information similar to the way a human can. A human can read a paragraph and distinguish between types of information, but our UiPath bots can't. Document Understanding integrates with artificial intelligence to interpret information within that.
The newer versions of Document Understanding can integrate with ChatGPT or any generative AI tools so that it can better interpret the information autonomously, and we don't need to create the taxonomy or classify the documents. We only need to give a prompt and input the document.
It will read documents similar to the way a human would. Let's use a contract as an example. You want to extract data like the buyer, seller, property address, etc. It will take the information from the document and give it to you. It can also scan for checkboxes and identify which ones are checked, but there are some limitations.
It uses a document object model to map which data is on what page of the document. For example, let's say the data you are interested in is on the third page of the document. The model knows where the data is, so it directly jumps to that particular page and extracts the information. The mapping is very perfect.
We always use attended processes because it's a good practice. The bot can do it without a human in the loop, but I would only do that if you are certain about which information you want to extract. If you're working with a handwritten document or signatures, you need a human in the loop to validate the data and help the machine learning component learn the difference between correct and incorrect information.
The time required for the validation process varies depending on the number of fields. For a small number, it only takes two or three minutes. When you have more fields, it may take a little longer to create and configure the document understanding model. You need to create the taxonomy, classifications, and model.
The validation process is easy. The Validation Station shows you the extracted data on one side and the document on the other, so you can easily scroll down and check if the data is accurate. You just need to click a checkbox. If you don't think it is fine, you have the option to add an exception. Based on that exception, you can create multiple conditions for how to address the same issue if it happens again.
Document Understanding is about 75-100 percent accurate depending on the type of document, and it increases as you train the model.
What needs improvement?
I would like to see more integration of artificial intelligence. That's being implemented, but it would be a massive improvement to the solution's document processing. If UiPath achieves intelligent document processing, it will be far better than anything on the market. There are currently some limitations with the fields that could be addressed using a GPT engine. With an integrated AI model, you wouldn't need to create your taxonomy. You would only need to provide some prompts, such as "What is the property name?" It will store that as a variable.
For how long have I used the solution?
I started using Document Understanding six months ago.
What do I think about the scalability of the solution?
In the community version, there is a limit on data extraction using a form-based extractor. There are limitations on digitization in the community version. You can do only 50 or so in one hour. The enterprise version can handle a larger volume of data, but we aren't dealing with huge amounts of data. We can still use multiple types. It allows you to scale with multiple types of extractors in the same document. If I'm confident in how the model is processing a particular field, it can be adopted into the regular business structure and reused.
Which solution did I use previously and why did I switch?
How was the initial setup?
I was involved in the deployment only as a developer. We created the taxonomy and the model for Document Understanding, then tested multiple cases with multiple documents. We see which extractor would be the best fit for a particular value. We can classify it according to the values we want and we can set up an accuracy also. We can set a confidence level for each variable, so the confidence is different for a regular extractor versus a complex one. I set the confidence level high on the regular extractor.
Initially, the deployment is somewhat complicated for a developer. However, it gets easier once you understand everything. We didn't need a consultant. I could complete the job by myself. It isn't rocket science. UiPath Academy has a free course on Document Understanding. Anyone can use it for free.
What's my experience with pricing, setup cost, and licensing?
We use the free community version. Anybody can use it, but it has some subtle limitations. The enterprise license gives you far better results without limitations.
Document Understanding can handle handwriting and signatures in most cases. The community version limits handwritten document processing, but it's enough for our needs and gives us the correct data every time.
Which other solutions did I evaluate?
I haven't worked with any other document processing solution besides UiPath. I researched some tools, but Document Understanding seemed like the best fit for me, so I used it.
What other advice do I have?
I rate UiPath eight out of 10. I deduct two points because creating the configurations can be time-consuming.
Which deployment model are you using for this solution?
Public Cloud
Disclosure: PeerSpot contacted the reviewer to collect the review and to validate authenticity. The reviewer was referred by the vendor, but the review is not subject to editing or approval by the vendor.
Last updated: Oct 2, 2023
Flag as inappropriateAccount Chief Technologist at Peraton
Saves time with processes like document classification, data extraction and automation
Pros and Cons
- "The solution removes manual processes and reduces human dependency. It takes a lot of effort to go through each physical mail or email, extract the data and transfer it to Excel. However, the solution automates the process and works 24/7. The tool gives a complete package to process and understand documents. The valuable features include taxonomy modification, classification, workstation, etc. There are out-of-the-box features like ML models which you can custom build. We have saved time with UiPath Document Understanding. We have seen a 50 percent improvement in scanning processes. Compared to humans, the tool runs 24/7. The human error rate has also been reduced. Our human error rate is five percent now compared to the previous 15 percent. UiPath Document Understanding has also freed up our staff who now spend more time on critical tasks."
- "There is room for improvement in UiPath Document Understanding's pricing. It is expensive for small clients. Currently, there is a big gap between the basic package and the 200,000 packages. There is no package in the middle for small agencies."
What is our primary use case?
I use the tool for a couple of my client projects. My clients receive physical mail and may need to scan data to run processes like automation on it. Another use case is document classification. The solution helps with processes like classification, data extraction, and automation.
What is most valuable?
UiPath removes manual processes and reduces human dependency. It takes a lot of effort to go through each physical mail or email, extract the data and transfer it to Excel. However, the solution automates the process and works 24/7.
It gives a complete package to process and understand documents. The valuable features include taxonomy modification, classification, workstation, etc. There are out-of-the-box features like ML models which you can custom build.
We have saved time with UiPath Document Understanding. We have seen a 50 percent improvement in scanning processes. Compared to humans, the tool runs 24/7. The human error rate has also been reduced. Our human error rate is five percent now compared to the previous 15 percent.
UiPath Document Understanding has also freed up our staff who now spend more time on critical tasks.
What needs improvement?
There is room for improvement in UiPath Document Understanding's pricing. It is expensive for small clients. Currently, there is a big gap between the basic package and the 200,000 packages. There is no package in the middle for small agencies.
For how long have I used the solution?
I have been working with the solution for more than five years. I started to work on the product when it was still under development.
What do I think about the stability of the solution?
I have not encountered any performance issues.
What do I think about the scalability of the solution?
The exact number of documents processed per client varies. However, it ranges between 1000-3000 per week. The documents processed are very less. We process 10-15 documents daily.
How are customer service and support?
UiPath Document Understanding's support is always ready and helpful.
How would you rate customer service and support?
Positive
How was the initial setup?
UiPath Document Understanding was easy to implement and put into production. The timeline can change when you create your ML model.
What was our ROI?
We have seen ROI with UiPath Document Understanding.
What other advice do I have?
The document format is mostly PDF and can be structured or semi-structured documents. We have not dealt with handwritten documents. Our real-time use case is for structured documents like emails and invoices. Most of the client documents go through without any errors. However, there is a five percent failure rate that needs to be considered since the document may contain unexpected data. 90 percent of documents go through it.
The solution handles signature-based documents. We are still working on that prototype. We faced issues with seals. It differs from department to department and state to state.
The tool's AL and ML features work fine for us. We leverage these features for driving licenses. AL and ML keep a check on document generation. UiPath Document Understanding has come up with an API-based document understanding model which we will leverage soon.
We implement human validation when we use anything new so that everything works as expected.
I would rate UiPath Document Understanding an eight out of ten.
Disclosure: I am a real user, and this review is based on my own experience and opinions.
Product Manager at a hospitality company with 51-200 employees
Good documentation understanding and helpful technical support with the capability to free up staff time
Pros and Cons
- "We can integrate document understanding with other systems and applications."
- "If there were more integrations with Veracode or the AWS server, so we don't have to completely transfer our data and keep data on our servers, that might increase security."
What is our primary use case?
We use the solution in pharmacy health care, and our role is to enable doctors so that they can set up a personalized clinic - everything a patient requires. We get information in the form of a document and we can break it down into sheets and JSON files, for example. We use a UiPath documentation tool.
How has it helped my organization?
Document understanding has helped us increase our efficiency and accuracy. We don't have to manually check data again and again.
After the first month, we discussed how the solution was benefiting us, and we decided to continue with it.
What is most valuable?
It helps with data and consistency. It helps us receive information and convert it so the systems we have in place can understand a problem and generate responses accordingly.
We've used it in one process where we received a patient's pharmaceutical documents from other sources that come in different formats. We receive the formats, convert the information into a standard format, and then process the information to provide information for insurance forms.
The average document size is not very large, likely 80-100 MBs. However, the total count of the patients is somewhere around 10,000.
We have 50% to 60% of clients directly onboarded via an insurance form. Therefore, we are provided with the exact form we need and can run a complete automation on that. There's no type of manual involvement there.
The format for setup is a great thing. Earlier, the tool that we used was pretty manual. In this case, it's a bit easier for our developers.
The solution can detect signatures to let us know that there's a signature there. You can construct tables or any other format of data based on pure text information.
They are employing an ML model for detection conversations. They are also trying to deploy a written-to-text conversion. They are convinced AMR systems will replace other manual work.
The main value of AI for us is to convert data formats from one type to another. We receive data stating two or more complex data points mixed later, for example, the license number and the serial date of operation for the doctors or the patient code; sometimes these things are mixed together. We want all those to be arranged. Their AI does the job very well.
We can integrate document understanding with other systems and applications. With it, we can simply write down a code to communicate with the ML model, for example, how to convert the data and which datasets to look for precisely in the documentation. We were able to communicate easily what would be the format of the PDF documents that we would be providing. The integration part and communication was the best aspect of the entire application.
We have Veracode integrated with it. We will do a manual check if we get a security flag where the data may be inconsistent. We usually get an alert like this once or twice a week. The human validation process usually takes an hour since we have to manually check the parameters. Before implementing the solution, the handling time before automating the process was pretty much the same. With this, we may have reduced it by half an hour. Also, previously, we'd have more manual interventions happening, maybe three or four times a day; however, now, with everything automated, that only happens one or two times a week. It's reduced the frequency by about half an hour on average.
Using the solution has freed up staff time. We've reduced our team size in regards to quality checking. We've reduced the amount of work by 40 to 50 hours a week.
What needs improvement?
UiPath's documentation tool is not great with converting handwriting to text, so we only used it for the conversion of insurance documents into other formats.
They could modulate the ML model in the future. When it comes to working with data and processing reports, we have to target the datasets we had earlier targeted and redefine the parameters, which takes a lot of time. If the ML model, at the time it is analyzing the data, could in itself provide the insights we will need for future reporting, that would be great. There needs to be better real-time analytics since we aren't getting the data for reporting until we go and seek it out.
If there were more integrations with Veracode or the AWS server, so we don't have to completely transfer our data and keep data on our servers, that might increase security.
For how long have I used the solution?
I've used the solution for a year or so.
What do I think about the stability of the solution?
The solution is good. It's very stable.
What do I think about the scalability of the solution?
It's not deployed across multiple departments. We have this deployed across one department. We have two developers working with the stream of data.
For small to medium firms, the solution scales well. However, if you are going for a global scale, you should develop your own models and not rely on outside models.
How are customer service and support?
Support is good. That said, sometimes they have problems understanding what we want to do with the data since we cannot provide the data in its raw format. We have to decrypt it. This makes it a bit harder. That's why we would like integration on our servers instead of theirs.
How would you rate customer service and support?
Positive
Which solution did I use previously and why did I switch?
We did use a different solution previously. We switched since the number of tags we were getting was pretty high. We had to do more manual interventions a lot more often. The parameters we used to communicate were also manual. It required setting up a decision tree in the whole of the document. A lot of the time, we would not know what the document type would look like. It required the developers to look at the documents, create a decision tree, and go from there. With UiPath, we don't need to do all that manual upfront work.
How was the initial setup?
I was a project manager, not a developer, deploying the solution. My understanding is the process was moderate. It was eight too easy or too complex.
The implementation involved discussing the work with the insurance firm. We explained we were moving from one system to another. Once we had that conversation, we received the documentation in the format we wanted.
Then, we looked at how we encrypted our data before sending it to UiPath servers. We did have a lot of compliance issues and had to be careful.
Once we came to the physical implementation, that was easy. Managing other stakeholders and their clients was the hardest part.
We had three developers from our team working on the deployment. It took us about 10 to 11 days to deploy.
Twice a week, maintenance is needed whenever there's a flag raised when data points do not match. We can simply ignore the solution and change the data file, or we can go in and see what is wrong with the file type and adjust it so that it doesn't happen again.
What about the implementation team?
We did not use any outside assistance beyond the help of UiPath's support team.
What was our ROI?
The ROI is pretty good. We did not do any calculation for ROI. However, the accuracy percentage and time reduction which we noted, have made us happy.
We originally noticed a time to value for UiPath within 10 to 12 days.
What's my experience with pricing, setup cost, and licensing?
The pricing is pretty fair. It is quote-based. Overall, it's fair. If you are a small firm looking to scale up, it is good. Enterprises should create their own ML model instead of relying on some outside product.
Which other solutions did I evaluate?
We looked at a few other options and did a few POCs. UiPath is able to sense and analyze a document and create a hierarchy for you. You can also create a manual code if you want something done differently. The only issue is we have to upload the information to UiPath servers, which may be a security issue.
What other advice do I have?
We're end-users, not integrators.
It's a good idea to have a call with the support team and managers and do a review to understand the solution to see if the product would work with your type of data. It's important to test it out, ideally using your own data.
I'd rate the solution nine out of ten.
Which deployment model are you using for this solution?
Private Cloud
Disclosure: PeerSpot contacted the reviewer to collect the review and to validate authenticity. The reviewer was referred by the vendor, but the review is not subject to editing or approval by the vendor.
Last updated: Nov 16, 2023
Flag as inappropriateTechnical Lead at Q3 Technologies
Helps reduce human error, saves staff time, and provides valuable OCR technology
Pros and Cons
- "OCR technology is undoubtedly the most valuable feature and the feasibility of integrating data processes with AI and machine learning models is fascinating."
- "The machine learning model needs improvement, as we receive more and more unstructured documents from clients that require a lot of manual validation."
What is our primary use case?
We use UiPath to automate invoice generation in our manufacturing process. One large project I worked on was for electricity bill payments. This project involved document processing, and I gained some experience with document processing and process mining. From there, we started using UiPath Document Understanding for the bulk of invoices we were receiving. We then had to put those invoices into the document processing model because they had a uniform structure, but there were also some handwritten notes and values in different places. So, we had to opt for document processing. Right now, we are developing a proof of concept for one of our government websites. This involves tender documents. We download and process the tender documents, extracting data such as the quotation, validity period, and other details, and putting it into a database.
We are processing documents in the hundreds using UiPath Document Understanding.
The standard document contains header information such as the company name, quoted value, quotation price, and expiration date. There are also tabular details regarding the items to be delivered. The tabular structure has headers, but checkboxes are not present in this particular use case. In addition to the header and tabular details, the document may also contain handwritten notes.
We have deployed UiPath Document Understanding on-premises but given the choice we always recommend the cloud because it includes more features.
How has it helped my organization?
UiPath Document Understanding eliminated the manual process of extracting data from 50 different websites each day.
Our customers' documents vary by website, but their structure is fairly uniform. As a result, we were able to process approximately 70-75 percent of the documents automatically with very good accuracy.
UiPath Document Understanding can identify and export signatures and handwriting from clear documents, using machine learning.
AI and machine learning feed the unprocessed raw data to some of the custom machine learning models. I have been working as a backend developer, so I have experience with machine learning as well. I tried with some of my own models, and it was clear that the customization of these models to our specific data requirements is very impressive.
UiPath Document Understanding's ability to integrate with all the systems and applications in our environment depends on the specific requirements of our use case. If it is generating a good return on investment, then I will consider using it for document processing. However, if my requirements can be met without using document processing, I will definitely choose to use simple OCR techniques instead. Traditional OCR engines can extract data from documents and place it into databases, where it can then be manipulated. However, this approach can be time-consuming and error-prone.
UiPath Document Understanding has helped our organization improve. It is especially useful when there is ambiguity in documents, which is a common real-life scenario. Inbuilt OCR engines are often unable to perform data inspection accurately in such cases. Whenever we have a large volume of documents to process and need to ensure high accuracy, UiPath Document Understanding is our first choice. One of the key benefits of UiPath Document Understanding is that it provides a dedicated model for document processing. This means that developers do not need to worry about other details and can focus solely on the task at hand. Additionally, UiPath Document Understanding integrates seamlessly with machine learning and AI models, which further enhances its capabilities.
Some of our customers were reluctant to switch over, and for a long time, they did everything manually, so their documentation was very outdated. As a result, we were required to manually validate 30 percent of the documents. The time to manually validate depends on each document. If two or three fields are mismatched, it does not take much time to correct them. However, if the entire document is showing errors, that will add time to the manual validation process.
It reduces the risk of human error and the time we spend processing documentation, freeing up our staff to work on other projects.
What is most valuable?
OCR technology is undoubtedly the most valuable feature and the feasibility of integrating data processes with AI and machine learning models is fascinating.
What needs improvement?
The identification and extraction of signatures is the most difficult part of the process, and there is room for improvement.
The machine learning model needs improvement, as we receive more and more unstructured documents from clients that require a lot of manual validation.
For how long have I used the solution?
I have been using UiPath Document Understanding for three years.
What do I think about the stability of the solution?
UiPath Document Understanding is stable.
What do I think about the scalability of the solution?
UiPath Document Understanding is scalable.
What's my experience with pricing, setup cost, and licensing?
I've seen many clients refuse to purchase the licensing when they see the pricing. They're quite impressed with the results, as the bot does so much work in less time with accuracy. However, when it comes to pricing, I've seen clients refuse to spend that much on the licensing cost for UiPath Document Understanding.
On a scale of one to ten with ten being the most expensive, I rate UiPath Document Understanding an eight on cost.
What other advice do I have?
I would rate UiPath Document Understanding eight out of ten.
I definitely recommend UiPath Document Understanding to anyone who is trying to do any kind of document automation. In fact, I have some friends who are working on an RPA project using UiPath, and we have been discussing it. I recommended Document Understanding when it first came out, and I think they have been using it for the project.
Which deployment model are you using for this solution?
Public Cloud
If public cloud, private cloud, or hybrid cloud, which cloud provider do you use?
Microsoft Azure
Disclosure: My company has a business relationship with this vendor other than being a customer: consultant
Last updated: Sep 29, 2023
Flag as inappropriate
Buyer's Guide
Download our free UiPath Document Understanding Report and get advice and tips from experienced pros
sharing their opinions.
Updated: April 2024
Product Categories
Intelligent Document Processing (IDP)Popular Comparisons
ABBYY Vantage
HyperScience
Tungsten TotalAgility
OpenText Intelligent Capture
Hyland Brainware
Datamatics TruCap+
Buyer's Guide
Download our free UiPath Document Understanding Report and get advice and tips from experienced pros
sharing their opinions.
Quick Links
Learn More: Questions:
- What is the difference between Robotic Process Automation (RPA) and Intelligent Process Automation (IPA)?
- When evaluating ABBYY, what aspect do you think is the most important to look for?
- Would you choose to produce an intelligent automation solution or develop plugins for the big five (SAP, Oracle, IBM, Microsoft and Google)?
- What is the best intelligent document processing solution?
- What is an intelligent document processing solution?
Thank you for your valuable review Biswajeet.