StreamSets Valuable Features
SS
SrinivasanSankar
Enterprise Solutions Architect at a energy/utilities company with 1,001-5,000 employees
StreamSets is GUI-based and takes care of load balancing. It allows a hybrid installation approach, rather than being completely cloud-based or on-premises. Additionally, StreamSets provides good enterprise support with a quick turnaround.
View full review »The best thing about StreamSets is its plugins, which are very useful and work well with almost every data source. It's also easy to use, especially if you're comfortable with SQL. You can customize it to do what you need. Many other tools have started to use features similar to those introduced by StreamSets, like automated workflows that are easy to set up.
View full review »SS
SrinivasanSankar
Enterprise Solutions Architect at a energy/utilities company with 1,001-5,000 employees
The types of the source systems that it can work with are quite varied. There are numerous source systems that it can work with, e.g., a SQL Server database, an Oracle Database, or REST API. That is an advantage we are getting.
The most important feature is the Control Hub that comes with the DataOps Platform and does load balancing. So, we do not worry about the infrastructure. That is a highlight of the DataOps platform: Control Hub manages the data load to various engines.
It is quite simple for anybody who has an ETL or BI background and worked on any ETL technologies, e.g., IBM DataStage, SAP BODS, Talend, or CloverETL. In terms of experience, the UI and concepts are very similar to how you develop your extraction pipeline. Therefore, it is very simple for anybody who has already worked on an ETL tool set, either for your data ingestion, ETL pipeline, or data lake requirements.
We use StreamSets to load into AWS S3 and Snowflake databases, which are then moved forward by Power BI or Tableau. It is quite simple to move data into these platforms using StreamSets. There are a lot of tools and destination stages within StreamSets and Snowflake, Amazon S3, any database, or an HTTP endpoint. It is just a drag-and-drop feature that is saving a lot of time when rewriting any custom code in Python. StreamSets enables us to build data pipelines without knowing how to code, which is a big advantage.
The data resilience feature is good enough for our ETL operations, even for our production pipelines at this stage. Therefore, we do not need to build our own custom framework for it since what is available out-of-the-box is good enough for a production pipeline.
StreamSets data drift feature gives us an alert upfront so we know that the data can be ingested. Whatever the schema or data type changes, it lands automatically into the data lake without any intervention from us, but then that information is crucial to fix for downstream pipelines, which process the data into models, like Tableau and Power BI models. This is actually very useful for us. We are already seeing benefits. Our pipelines used to break when there were data drift changes, then we needed to spend about a week fixing it. Right now, we are saving one to two weeks. Though, it depends on the complexity of the pipeline, we are definitely seeing a lot of time being saved.
View full review »Buyer's Guide
StreamSets
June 2025
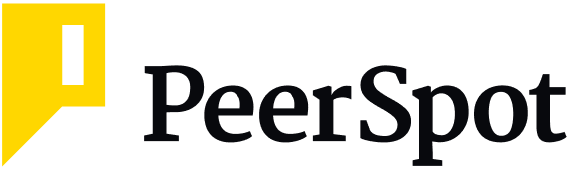
Learn what your peers think about StreamSets. Get advice and tips from experienced pros sharing their opinions. Updated: June 2025.
860,632 professionals have used our research since 2012.
The most valuable features are the option of integration with a variety of protocols, languages, and origins. I used the solution to integrate with Kafka and send emails and different types of data feeds. The UI is quite nice and easy to use, making it a simple task for me to find the processes, execute them, and achieve my goals.
The things I like about StreamSets are its
- overall user interface
- efficiency
- product features, which are all good.
Also, the scheduling within the data engineering pipeline is very much appreciated, and it has a wide range of connectors for connecting to any data sources like SQL Server, AWS, Azure, etc. We have used it with Kafka, Hadoop, and Azure Data Factory Datasets. Connecting to these systems with StreamSets is very easy. You just need to configure the data sources, the paths and their configurations, and you are ready to go.
It is very efficient and very easy to use for ETL pipelines. It is a GUI-based interface in which you can easily create or design your own data pipelines with just a few clicks.
As for moving data into modern analytics systems, we are using it with Microsoft Power BI, AWS, and some on-premises solutions, and it is very easy to get data from StreamSets into them. No hardcore coding or special technical expertise is required.
It is also a no-code platform in which you can configure your data sources and data output for easy configuration of your data pipeline. This is a very important aspect because if a tool requires code development, we need to hire software developers to get the task done. By using StreamSets, it can be done with a few clicks.
View full review »I have used Data Collector, Transformer, and Control Hub products from StreamSets. What I really like about these products is that they're very user-friendly. People who are not from a technological or core development background find it easy to get started and build data pipelines and connect to the databases. They would be comfortable like any technical person within a couple of weeks. I really like its user-friendliness. It is easy to use. They have a single snapshot across different products, which is very helpful to learn and use the product based on your use case.
Its interface is very cool. If I'm using a batch project or an ETL, I just have to configure appropriate stages. It is the same process if you go with streaming. The only difference is that the stages will change. For example, in a batch, you might connect to Oracle Database, or in streaming, you may connect to Kafka or something like that. The process is the same, and the look-and-feel is the same. The interface is the same across different use cases.
It is a great product if you are looking to ramp up your teams and you are working with different databases or different transformations. Even if you don't have any skilled developers in Spark, Python, Java, or any kind of database, you can still use this product to ramp up your team and scale up your data migration to cloud or data analytics. It is a fantastic product.
View full review »There are two features that are most valuable for us. One is the Control Hub and the other is the Data Collector. With Data Collector, data migration has become much easier for us.
Also, the ETL capabilities are very useful for us. We extract and transform data from multiple data sources, into a single, consistent data store, and then we put it in our systems. We typically use it to connect our Apache Kafka with data lakes. That process is smooth and saves us a lot of time in our production systems.
We use the platform to incorporate modern analytics as well. That is one of our main use cases. It integrates well with our requirements. It is quite easy to move data into these analytics platforms using StreamSets because there are minimal coding requirements. The built-in applications and systems allow us to do it with ease. A first-time user could easily do it.
If there were coding requirements, it would take three or four extra resources to get things done. That aspect is very important for us. It saves us money by not needing coding manpower.
In addition, the system's data drift resilience is very effective and efficient. On our particular team, it has reduced the time it takes to fix data drift breakages by 10 to 12 man-hours per week.
View full review »MI
Mwase Isaranya
Software Engineer at Soft Hostings Limited
What I love the most is that StreamSets is very light. It's a containerized application. It's easy to use with Docker. If you are a large organization, it's very easy to use Kubernetes.
It has a very easy and user-friendly interface. It only takes a few days for new developers to start and deploy their first pipeline. It provides an easy and powerful integrated environment with different platforms such as Kafka, Salesforce, Oracle Database, REST API, etc. The user interface is a powerful feature of StreamSets.
View full review »The ability to have a good bifurcation rate and fewer mistakes is valuable. In the scenario we had, when we had to bifurcate the data, we did not completely cut the data. We made a different route for one set of data, which went into a different operating system. There was also a complete set of data along with the original data that got cut, which once again went through the filtration process, and in this way, it kept on happening. Different solutions that were in place were not providing this feasibility. With the other solutions that we were using earlier, we had to reuse the data again and again from the start. It was a time-taking process.
Their support system was pretty good. When we were setting up the bifurcation protocols that we wanted to set up, we had a few support calls with them, and those were really helpful.
One of the things I like is the data pipelines. They have a very good design. Implementing pipelines is very straightforward. It doesn't require any technical skill.
We have also integrated it with Kafka messaging and it is not complex to do. It is really so easy to connect or integrate with data interfaces. And moving data into analytics platforms using StreamSets is easy. It doesn't require any coding, meaning your can transfer or move data into data payloads without coding skills. It's a good move, for someone in the beginning, who doesn't have any knowledge because it's quite easy.
View full review »The best feature that I really like is the integration. The software can be integrated with Azure Keyvault or AWS Secrets Manager, as well as scheduling. It is very easy to schedule an event, which is much easier than I expected through StreamSets. The solution is also fast at determining pipelines. Additionally, I like that StreamSets has many components, such as sources, processes, execution, and other useful elements that I need to plan.
For me, the most valuable features in StreamSets have to be the Data Collector and Control Hub, but especially the Data Collector. That feature is very elegant and seamlessly works with numerous source systems.
Also, the intuitive canvas for designing all the streams in the pipeline, along with the simplicity of the entire product are very big pluses for me. The software is very simple and straightforward. That is something that is needed right now.
Apart from that, the user interface of StreamSets is very good. It's very user-friendly and very appealing. Moving data into modern analytics platforms is a very straightforward procedure. There is no difficulty involved in it.
In addition, the ETL capabilities of StreamSets are also very useful for us. We are able to extract and transform data from multiple data sources into a single, consistent data store that is loaded into our target system.
I really appreciate the numerous ready connectors available on both the source and target sides, the support for various media file formats, and the ease of configuring and managing pipelines centrally. It's like a plug-and-play setup.
View full review »The most valuable features of StreamSets, for me, are the Data Collector and the Control Hub platform. They are both very straightforward to use and user-friendly. And with the Data Collector and Control Hub, we get canvas selection for designing all our pipelines, which is very intuitive and useful for us.
In fact, the entire user interface is very simple and the simplicity of creating pipelines is something that I like very much about it. The design experience is very smooth. A great thing about StreamSets is that it is a single, centralized platform. All our design-pattern requirements are met with a single design experience through StreamSets.
We can also easily build pipelines with minimal coding and minimal technical knowledge. It is very easy to start and very easy to scale as well. That is very important to me, personally, because I'm from a non-technical background. One of the most important criteria was for me to be able to use this platform efficiently.
Also, moving data to modern analytics platforms is very straightforward. That is why StreamSets is one of the top players in the market right now.
And one of the major advantages for us is the built-in functionality. StreamSets has a plethora of features that combine well with ETL.
View full review »The most valuable would be the GUI platform that I saw. I first saw it at a special session that StreamSets provided towards the end of the summer. I saw the way you set it up and how you have different processes going on with your data. The design experience seemed to be pretty straightforward to me in terms of how you drag and drop these nodes and connect them with arrows.
The most valuable feature is the pipelines because they enable us to pull in and push out data from different sources and to manipulate and clean things up within them.
We use StreamSets to connect to enterprise data stores, including OLTP databases and Hadoop. Connecting to them is pretty easy. It's the data manipulation and the data streaming that are the harder parts behind that, just because of the way the tool is written.
It's very effective in project delivery. This month, at the end of June, I will deploy all the integrations which I developed in StreamSets to production remit. The business users and customers are happy with the data flow optimizer from the SoPlex cloud. It all looks good.
Not many challenges are there in terms of learning new technologies and using new tools. We will try and do an R&D analysis more often.
Everything is in place and it comes as a package. They install everything. The package includes Python, Ruby, and others. I just need to configure the correct details in the pipeline and go ahead with my work.
The ease of the design experience when implementing batch streaming and ETL pipelines is very good. The streaming is very good. Currently, I'm using Data Collector and it’s effective. If I'm going to use less streaming, like in Java core, I need to follow up on different processes to deploy the core and connect the database. There are not so many cores that I need to write.
In StreamSets, everything is in one place. If you want to connect a database and configure it, it is easy. If you want to connect to HTTP, it’s simple. If I'm going to do the same with my other tools, I don’t need many configurations or installations. StreamSets' ability to connect enterprise data stores such as OLTP databases and Hadoop, or messaging systems such as Kafka is good. I also send data to both the database and Kafka as well.
You will get all the drives that you will need to install with the database. If you use other databases, you're going to need a JDBC, which is not difficult to use.
I'm sending data to different CDP URL databases, cloud areas, and Azure areas.
StreamSets' built-in data drift resilience plays a part in our ETL operations. We have some processors in StreamSets, and it will tell us what data has been changed and how data needs to be sent.
It's an easy tool. If you're going to use it as a customer, then it should take a long time to process data. I'm not sure if in the future, it will take some time to process the billions of records that I'm working on. We generally process billions of records on a daily basis. I will need to see when I work on this new project with Snowflake. We might need to process billions of records, and that will happen from the source. We’ll see how long it needs to take and how this system is handling it. At that point, I’ll be able to say how effectivly StreamSets processes it.
The Data Collector saves time. However, there are some issues with the DPL.
StreamSets helped us break down data silos within our organizations.
One advantage is that everything happens in one place, if you want to develop or create something, you can get those details from StreamSets. The portal, however, takes time. However, they are focusing on this.
StreamSets' reusable assets have helped to reduce workload by 32% to 40%.
StreamSets helped us to scale our data operations.
If you get a request to process data for other processing tools, it might take a long time, like two to three hours. With this, I can do it within half an hour, 20 or 30 minutes. It’s more effective. I have everything in one place and I can configure everything. It saves me time as it is so central.
View full review »It is a pretty easy tool to use. There is no coding required. StreamSets provides us a canvas to design our pipeline. At the beginning of any project, it gives us a picture, which is an advantage. For example, if I want to do a data migration from on-premise to cloud, I will draw it for easier understanding based on my target system, and StreamSets does exactly the same thing by giving us a canvas where I can design our pipeline.
There are a wide range of available stages: various sources, relational sources, streaming sources. There are various processes like to transform the source data. It is not only to migrate data from source to destination, but we can utilize different processes to transform the data. When I was working on the healthcare project, there was personal identification information on the personal health information (PHI) data that we needed to mask. We can't simply move it from source to destination. Therefore, StreamSets provides masking of that sensitive data.
It provides us a facility to generate schema. There are different executors available, e.g., Pipeline Finisher executor, which helps us in finishing the pipeline.
There are different destinations, such as S3, Azure Data Lake, Hive, and Kafka Hadoop-based systems. There are a wide range of available stages. It supports both batch and streaming.
Scheduling is quite easy in StreamSets. From a security perspective, there is integration with keywords, e.g., for password fetching or secrets fetching.
It is pretty easy to connect to Hadoop using StreamSets. Someone just needs to be aware about the configuration details, such as which Hadoop cluster to connect and what credentials will be available. For example, if I am trying with my generic user, how do I connect with the Hadoop distributed system? Once we have the details of our cluster and the credential, we can load data to the Hadoop standalone file system. In our use case, we collected data from our RDBMS sources using JDBC Query Consumer. We queried the data from the source table, captured that data, and then loaded the data into the destination Hadoop distributed file system. Thus, configuration details are required. Once we have the configuration details, i.e., the required credentials, we can connect with Hadoop and Hive.
It takes care of data drift. There are certain data rules, matrix rules, or capabilities provided by StreamSets that we can set. So, if the source schema gets deviated somehow, StreamSets will automatically notify us or send alerts in automated fashion about what is going wrong. StreamSets also provides Change Data Capture (CDC). As soon as the source data is changed, it can capture that and update the details into the required destination.
StreamSets Transformer is a good feature because it helps you when you are developing applications and when you don't want to write a lot of code. That is the best feature overall. They really help you to come up with a solution more quickly. The Transformer logic is very easy, as long as you understand the concept of what you intend to develop. It doesn't require any technical skills.
The overall GUI and user interface are also good because you don't need to write complex programming for any implementation. You just drag and configure what you want to implement. It's very easy and you can use it without knowing any programming language.
The design experience is much easier when you want to integrate other systems and tools and make them work in a particular format. It helps you improve the topologies. You can view the status of all the pipelines you have developed and monitor them.
Connecting to enterprise data stores is also very easy, as is monitoring and managing things in one place.
View full review »It is really easy to set up and the interface is easy to use.
We found it pretty easy to transform data.
The online documentation is pretty good.
View full review »The ease of configuration for pipes is amazing. It has a lot of connectors. Mainly, we can do everything with the data in the pipe. I really like the graphical interface too. It's pretty nice.
View full review »Buyer's Guide
StreamSets
June 2025
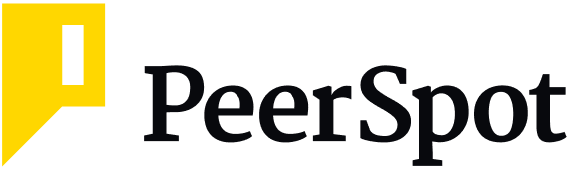
Learn what your peers think about StreamSets. Get advice and tips from experienced pros sharing their opinions. Updated: June 2025.
860,632 professionals have used our research since 2012.